The development and publication of artificial intelligences like OpenAI’s ChatGPT has attracted a lot of interest not only among scientists and (software) engineers but reaches deep into our diverse society. This technology provides obvious advantages but also causes undisputable issues and challenges. Next to ethical dilemmas, the tricky questions related to intellectual property and revolutionizing many jobs or making them obsolete, the immense energy consumption and corresponding emission of carbon dioxide required for training AI systems is widely discussed (Markovic et al., Nat. Rev. Phys 2, 2020).
Following Maha’s earlier blog post (“Neuromorphic Computing: A Brief Explanation” posted in December 2022, we recommend reading that one before delving into this second part) dealing with the von Neumann bottleneck and the basic functionalities of neurons and synapses, we will build up and try to exemplify in further detail, how magnetic systems can provide solutions to the imposed challenges. Therefore, the research field of spintronics tries to collaborate interdisciplenarily with researchers investigating the highly intriguing way the human brain works, and may perhaps also contribute to reducing the climate impact of this technological revolution which is on its way – for better or worse.
Conventional electronics uses „1“ and „0“ as elementary building blocks to save and compute information, also when emulating the potential in a neuron or the weight of a synapse. For instance, this implies that in order to have 32 different synaptic weight values accessible we need five elementary building blocks that can save one 1 or 0 to make up a number between 1 and 32 in the binary system. If instead we could find an alternative elementary building block, which has intrinsically 32 or more states available that is equally sized and may change its state at equal power and time scales, we could significantly improve our computing systems. Let us look at an example provided by spintronics for such an application:
A so-called domain wall separates regions with opposing magnetizations (blue and red in the image below) in a magnetic material. Such a wall can be moved by electric currents into the direction of the electrons. This implies that by using charge currents, we can change the magnetic state of the material. Now, a device can be designed such that the position of the domain wall determines the measured resistance. This is achieved by so called magnetic tunnel junctions, which we are not going to describe in further details here. As the resistance of such a device can vary between many values, depending on the position of the domain wall, we can interpret this as a synaptic weight where not only 1 and 0 but many more values are possible. Ideally, the domain wall can stop anywhere within a material making numerous states available. In real devices, such domain walls will prefer to locate around imperfections in the crystal, such as impurities („wrong atom“) or vacancies („missing atoms“). By engineering a shape geometrically to provide „prefered locations“ for such domain walls, the number of accessible state can be controlled. In the work by Leonard et al. (Adv. Electron. Mater. 2022, 8, 2200563), notches at the boundary of the magnet provide such locations. Thereby, an artificial synapse is designed that can be driven and read out at low energies and fast.

The location of a domain wall can also be used as a neuron potential such that this device could emulate a neuron. For this, a mechanism needs to be established that drives the wall back to one end in the absence of inputs, i.e. electric currents. One way to achieve this is by implementing a thickness gradient in the magnetic layer. Now, if a lot of currents accumulate within short enough times, the domain wall is driven across the device to the other end and the measured output value should be significantly changed only when it the wall reaches its (non-equilibrium) end. This can be engineered by the location of the read out sensors. In this way, simple magnetic devices can be used as both synapses and neurons.
Depending on the materials used in the fabrication process, the desired algorithms, energy footprint, data density and speed, various advantages and disadvantages emerge which need to be quantified and better understood and improved by spintronics researchers and engineers. It is being emphasized that conventional electronics is already performing on a high level and it poses quite a challenge to compete with that technology. Replacing conventional electronics entirely with a new system based on magnets or some other physical system, is very unlikely. However, such systems can fill gaps and perform particular subtasks in bigger computational problems, that conventional electronics are not highly suited for.
Another property of brain inspired networks that is hard to reproduce in conventional electronics is the high interconnectivity between different neuron layers. Some ten thousands of connecting synapses are typical in brains but very hard to implement in electronics.
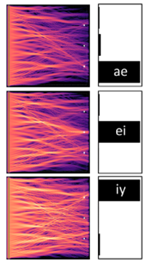
Papp et al. (Nat Commun 12, 6422, 2021) therefore demonstrate how to use a magnetic system in which the magnets are by default „talking to each other“ via their magnetic interaction and train the magnetic excitations of this system (so-called spin waves, which can be imagined similar to water waves) to tell apart different spoken vowels. Roughly, this can be imagined from the figure above in the following way: We have a plane of many little magnets (imagine a pool of water that experiences waves, that can be high or low just like the the magnetization can point upward or downward) represented by each of the three squares on top of each other. On the left the vowels are injected to the system as a high-frequency signal that excites the little magnets. If that sounds too crazy, think of a boat that can drive up and down on the left boundary of the pool at low, intermediate or high speed. The level of response (intensity of resulting water waves) is illustrated by the colors. The brighter the color the more the little magnets forward the information. The magnetic system can be trained by the implementation of some „fixed guiding magnets“ to redirect the incoming signals differently depending on what signal i.e., which vowel, was input. Perhaps you may think of buoys or obstacles in the water, that redirect the water waves. Thus, brighter color can be seen in the top, center or bottom part on the right side, where the signal can be read out again at one of the three white dots. Depending on which dot received the largest signal, the system recognized a different vowel (or a different speed of the boat).
This is an example (with a highly simplifying analogy of water waves) of how magnets can solve problems in a more elegant way by exploiting the wave nature of magnets than only implementing a lot of wires/connections with the conventional electronics.
As I am aware that some parts of this post may occur confusing and not intuitive right away, please do not hesistate to reach out to me, in case that you are interested to learn more of this emerging field of spintronics: marco.hoffmann@mat.ethz.ch .